Note
Go to the end to download the full example code
Timepicker
Following example showcases the results of different timepicking methods.
For more informations, please refer to the functions documentation (vallenae.timepicker
).
Read waveform from tradb
Prepare plotting with time-picker results
def plot(t_wave, y_wave, y_picker, index_picker, name_picker):
_, ax1 = plt.subplots(figsize=(8, 4), tight_layout=True)
ax1.set_xlabel("Time [µs]")
ax1.set_ylabel("Amplitude [mV]", color="g")
ax1.plot(t_wave, y_wave, color="g")
ax1.tick_params(axis="y", labelcolor="g")
ax2 = ax1.twinx()
ax2.set_ylabel(f"{name_picker}", color="r")
ax2.plot(t_wave, y_picker, color="r")
ax2.tick_params(axis="y", labelcolor="r")
plt.axvline(t_wave[index_picker], color="k", linestyle=":")
plt.show()
Hinkley Criterion
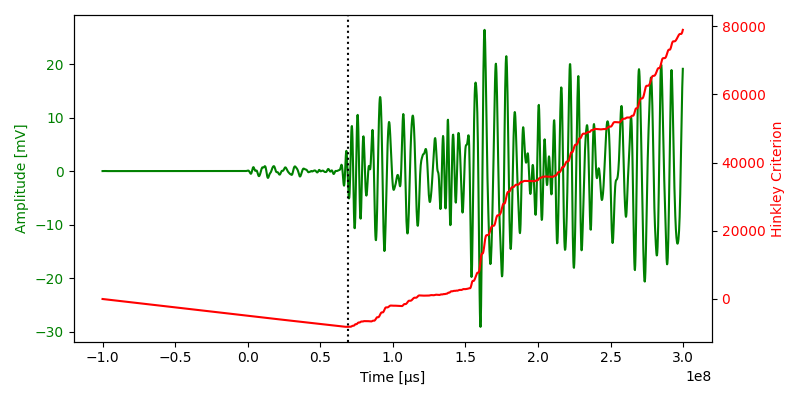
The negative trend correlates to the chosen alpha value and can influence the results strongly. Results with alpha = 50 (less negative trend):
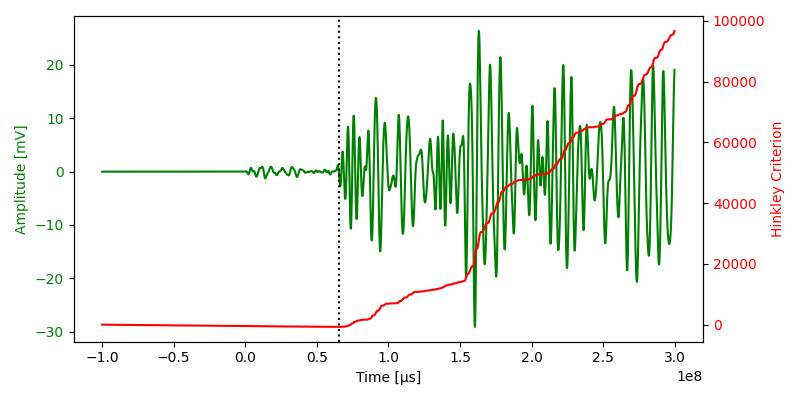
Akaike Information Criterion (AIC)
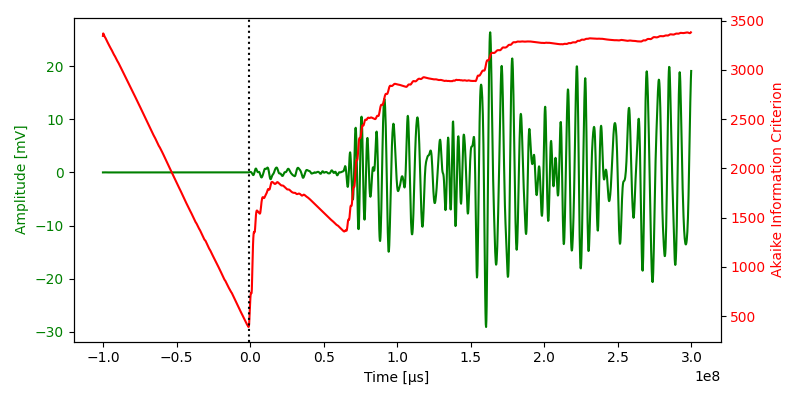
Energy Ratio
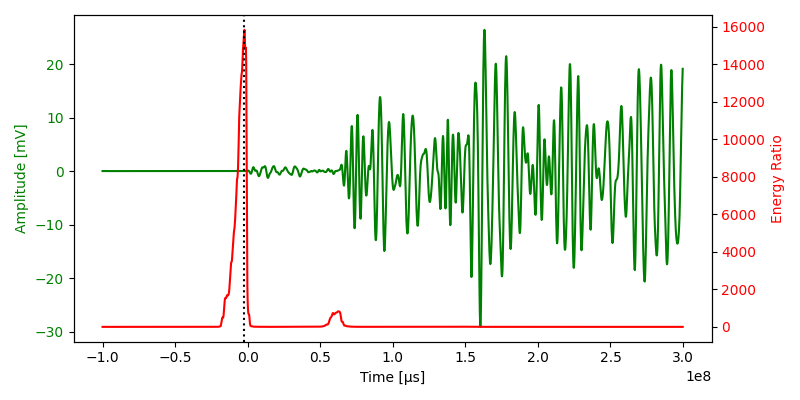
Modified Energy Ratio
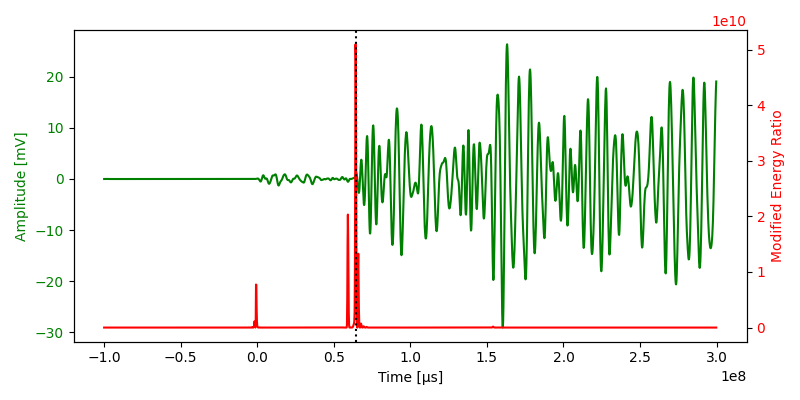
Performance comparison
All timepicker implementations are using Numba for just-in-time (JIT) compilations. Usually the first function call is slow, because it will trigger the JIT compiler. To compare the performance to a native or numpy implementation, the average of multiple executions should be compared.
def timeit(func, loops=100):
time_start = time.perf_counter()
for _ in range(loops):
func()
return 1e6 * (time.perf_counter() - time_start) / loops # elapsed time in µs
timer_results = {
"Hinkley": timeit(lambda: vae.timepicker.hinkley(y, 5)),
"AIC": timeit(lambda: vae.timepicker.aic(y)),
"Energy Ratio": timeit(lambda: vae.timepicker.energy_ratio(y)),
"Modified Energy Ratio": timeit(lambda: vae.timepicker.modified_energy_ratio(y)),
}
for name, execution_time in timer_results.items():
print(f"{name}: {execution_time:0.3f} µs")
plt.figure(figsize=(8, 3), tight_layout=True)
plt.bar(timer_results.keys(), timer_results.values()) # noqa: SIM911
plt.ylabel("Time [µs]")
plt.show()
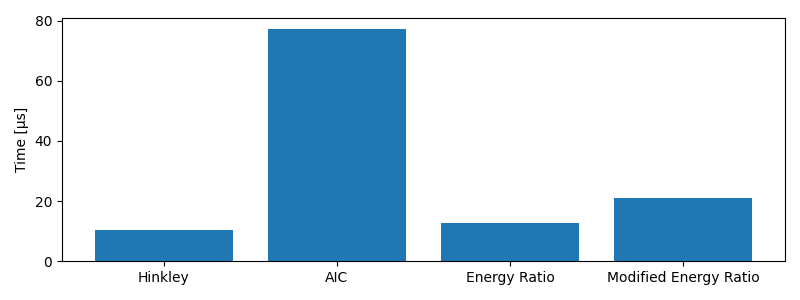
Hinkley: 10.551 µs
AIC: 77.192 µs
Energy Ratio: 12.600 µs
Modified Energy Ratio: 20.961 µs
Total running time of the script: (0 minutes 3.184 seconds)